The New Wave of AI in Healthcare
In the healthcare field, artificial intelligence has the potential to improve everything from workflow efficiency to patient outcomes.
By Stephen Treffinger
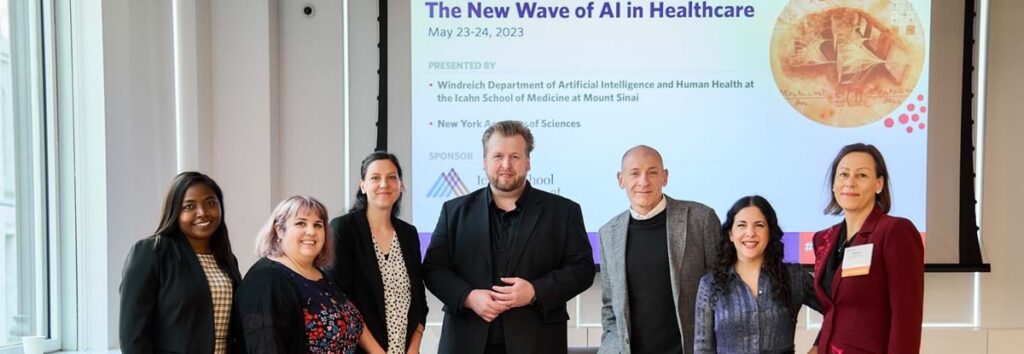
Members of the Organizing Committee. (From left to right) Affiliated with the Mount Sinai Health System: Christina Virgo, Esq., Sara Roncero-Menendez, Silke Muehlstedt, PhD, Thomas Fuchs, Dr. sc., Marc Kaplan; Affiliated with The New York Academy of Sciences: Melanie Brickman Borchard, PhD, MSc, Barbara Knappmeyer, PhD
Appearing on the front page of news outlets nearly every day, artificial intelligence (AI) is already transforming the world — and doing so at a staggering pace. In the healthcare field, it has the potential to improve everything from workflow efficiency to patient outcomes. But sifting through the hype can be a tremendous challenge.
The Windreich Department of Artificial Intelligence and Human Health at the Icahn School of Medicine at Mount Sinai and the New York Academy of Sciences hosted the ‘New Wave of AI in Healthcare’ symposium as a “call to action” — bringing together experts and leaders across the field to tackle this challenge through innovation, exchange, and collaboration.
Over the course of two days, May 23 and 24, 2023, researchers, academics, and industry leaders presented and discussed innovative research and clinical solutions with the potential to advance the capabilities of AI and to better serve patients and clinicians from diagnostics to long-term care. In their opening remarks, Nicholas Dirks, President and CEO of The New York Academy of Sciences, and Dr. Dennis Charney, Dean of the Icahn School of Medicine and President for Academic Affairs for the Mount Sinai Health System, challenged attendees to identify ways to utilize the enormous amounts of health data available to help predict, prevent, and develop more robust treatments for disease.
“Today, patients are dying not because of AI, but because of the lack of it,” stated Thomas J. Fuchs, Dr. Sc, Dean for AI and Human Health at Mount Sinai, lead member of the scientific organizing committee in opening the symposium, Dr. Fuchs further emphasized that although machine learning has already led to significant achievements across the field of healthcare, we are only at the beginning of an AI revolution in healthcare.
The two-day symposium featured sessions on foundational models that revolutionize diagnostics processes, infrastructural challenges to facilitate large-scale models and innovative deep learning solutions to deal with the petabytes of data generated in healthcare. Also, the symposium addressed ethical considerations for AI research in healthcare to eliminate bias and ensure its application is equitable as well as impactful.
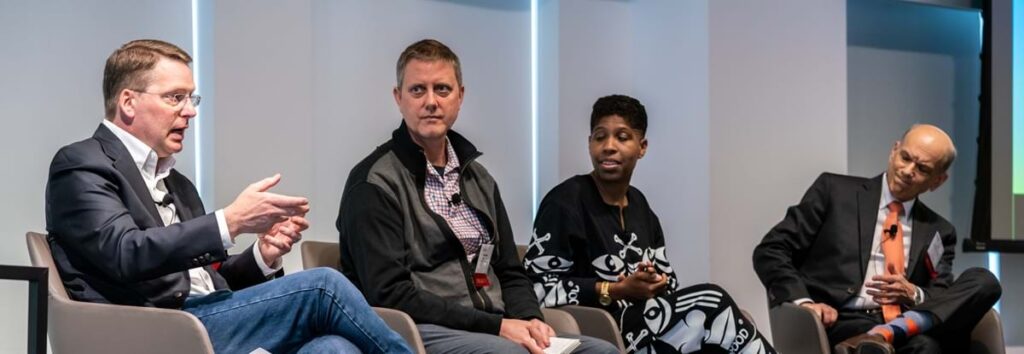
Panel Discussion: “Governance and Ethics for the Use of Large Healthcare Datasets for AI”. (From left to right) Panelists: Eric Lium, PhD, Mount Sinai Health System, Brandon D. Gallas, PhD, U.S. Food and Drug Administration, Emma Benn, DrPH, Mount Sinai Health System, Moderator: Eric Nestler, MD, PhD, Icahn School of Medicine at Mount Sinai
AI Fundamentals – Facts, Fictions, and Possibilities
Despite the immense hype around AI (as Dr. Fuchs remarked, “If you haven’t talked about ChatGPT, you’re probably living under a rock.”) and excitement in the start-up scene (including 14,000 healthcare startups in the AI realm), the reality is not quite as dramatic. There are only a few AI applications that are currently being used in the clinical setting to benefit patients. “While the FDA cleared hundreds of systems in radiology, in pathology, for example, there’s one single system that has proven to be safe and effective.”
Dr. Fuchs stated that AI gives us the possibility to truly democratize access to healthcare for the first time in history. “The AI we’re developing here and you’re developing at your fabulous institutions can be used in community clinics throughout the U.S. and throughout the world.”
The fact that clinicians are burned out and leaving practices has, according to several of the speakers, resulted in a deep crisis in healthcare. But AI is able to help combat this trend by automating workflows. One of the keynote speakers at the symposium, David C. Rhew, MD, Global Chief Medical Officer and VP of Healthcare at Microsoft, presented developments in Ambient Clinical Intelligence, which can, among other things, capture clinician-patient conversations and bring the information into the medical record.
“We have an ability now to pull data about individuals from every aspect. We can look at the perspective of what EHR [Electronic Health Record] data we have, what genomics data we have, and real-time data collection through remote monitoring.” This creates a 360-degree view of a person and one that changes as they evolve. “Now imagine having that at a population level,” he says. “That’s where the real power comes.”
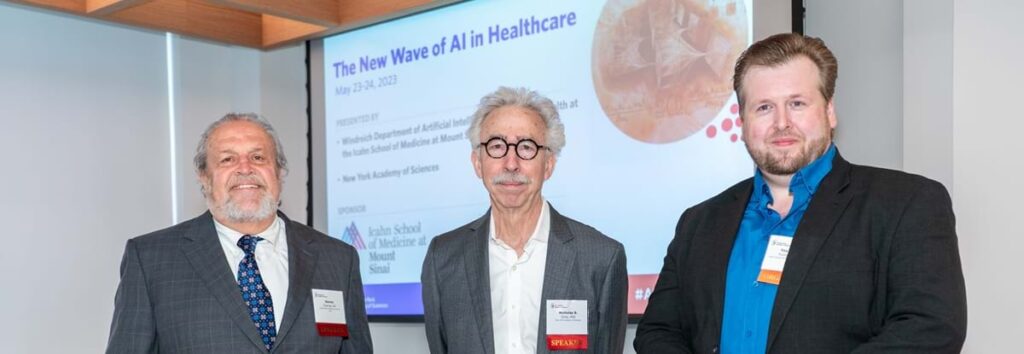
(From left to right) Dennis S. Charney, MD, Anne and Joel Ehrenkranz Dean of the Icahn School of Medicine at Mount Sinai, President for Academic Affairs, Mount Sinai Health System
Nicholas B. Dirks, PhD, President and Chief Executive Officer, The New York Academy of Sciences
Thomas J. Fuchs, Dr.sc., Co-Director of the Hasso Plattner Institute for Digital Health at Mount Sinai, Dean of Artificial Intelligence and Human Health, and Professor of Computational Pathology and Computer Science at the Icahn School of Medicine at Mount Sinai
AI for Healthcare and Life Sciences – Accelerated Discovery
Will more data and data-driven models yield better patient outcomes? This was a central theme throughout the symposium, which featured speakers utilizing foundational models in research and clinical diagnostic support tools to create deep learning models across heterogenous data modalities to improve patient outcomes.
The conference’s second keynote was delivered by Jianying Hu, PhD, from IBM Research and also an adjunct professor at the Icahn School of Medicine at Mount Sinai. In addition to IBM’s use of AI to drive accelerated discovery, she discussed what it means to go beyond large language models to apply foundational models to healthcare and life sciences research.
“In our view, [it’s] really all about enabling the journey from data to impact. AI can be used to help with the development of new molecular entities through novel generative methods, as well as computational screening tools [that] can be used to also identify new indications for drugs that are already approved,” says Dr. Hu. In addition to drug repurposing, AI is critical, she says, for being able to identify multimodal biomarkers.
From the Laboratory to Practice: Clinical Applications of AI
Moving from the theoretical to the practical and harnessing the full power of AI will require a change in approach, especially as it pertains to data: how much of it is required, how it needs to be treated, and how to maximize its effects. Abundant medical data is playing an important role, as precision medicine tries to determine the right treatment for the right patient at the right time.
“When we think about precision medicine and AI in medicine, we think about the health state of a patient and how we can model that health state of a patient,” says Gunnar Rätsch, PhD, ETH Zürich, who is currently conducting research as a visiting scientist within Mount Sinai’s AI Department.
Evidence of the health state comes from heterogeneous data modalities such as EHRs, pathology images, genomic profiles, drugs, and mobile health data. All of this data will have to be integrated into the computational representation to assess the true state of health of a patient. This requires new advances in AI approaches to exploit the specifics in the medical data, which requires genuine partnership between clinicians and machine learning/AI researchers.
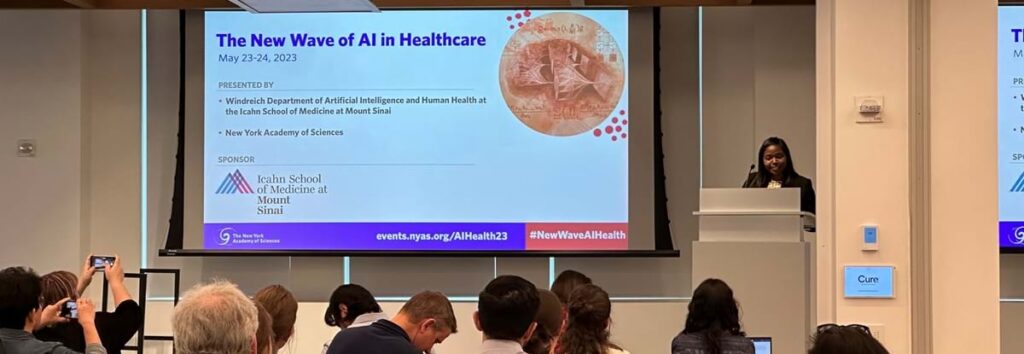
Christina Virgo, Esq., Director of Operations Hasso Plattner Institute for Digital Health at Mount Sinai
The Impact of the New Era of Large-scale Deep Learning Models
Increasing the speed by which researchers can process and analyze vast amounts of data is an ongoing challenge in AI. The exponential growth of healthcare data, genomics, electronic records, and imaging can overload systems and slow the path to progress.
But processing capacity and speed aren’t the only issues. Another challenge is to better merge clinical medicine and data science, the two being mutually beneficial. Anthony Chang, MD, from the Children’s Hospital of Orange County, thinks this is an essential—albeit largely absent—duality. There are too few people who are educated on both sides of the equation.
Dr. Chang also sees the need for a shift to a new paradigm of databases, i.e. graph databases. “These are more three-dimensional and much more accommodating of the complexities of healthcare data. […] I can’t imagine we’re going to get a lot more dividends using deep learning healthcare without a change in how we look at databases, which is relational databases.”
In the detection of breast cancer, to cite one example, having very large scan sizes is advantageous, but dealing with these enormous images efficiently can be problematic due to the amount of memory in the GPU and other factors. Krysztof J. Geras, PhD, NYU Langone Health, discussed the particulars of multiple instance learning. “We have this ability to indicate those parts of the image that are important. We can actually look at this image with greater detail with a higher capacity network, but just at a certain region of it, and then we can fuse this information. And that works amazingly well.”
AI at Mount Sinai
The Mount Sinai Health System is dedicated to leading an AI-driven transformation of healthcare through innovative research, pioneering clinical care, and personalization for each patient, aiming to have a wide-reaching impact on human health. In 2021, Mount Sinai established the Windreich Department of Artificial Intelligence and Human Health, the first department focused on AI and Human Health in any medical school in the United States.
As a leader in the AI in healthcare space, researchers at Mount Sinai are building an “intelligent fabric” of AI that will underpin all interdisciplinary efforts, combining AI, computer science, machine learning, and data science across the Health System to support every individual who comes through the hospital doors for care, as well as nurses, physicians, scientists, hospital operations and leadership,
Several Mount Sinai researchers presented their AI-integrated research during the symposium, highlighting the various ways in which this new technology can benefit researchers, clinicians, and patients from diagnosis to treatment, developing some of the most exciting advances in the field.
Highlights that were presented include:
- Ipek Ensari, PhD, who works with AI and machine learning combined with statistics in the field of women’s health, noted the possible link between indicators in the female reproductive system to diseases such as coronary artery disease, and stroke.
- Hayit Greenspan, PhD, focuses on AI in the medical imaging space. “We are developing a platform that provides tools for collection of the data, support of annotation of the data, and support of modeling that can be done to extract information that is useful from the imagery data.”
- Xiaosi Gu, PhD, focuses on the fast-growing sub-sector of mental health in health tech. “We need to both achieve a mechanistic understanding of the brain and of the mind at the algorithmic level and to use brain-related data to try to create predictive models.”
- John F. Crary, MD-PhD, is a neuropathologist and runs a research lab in neurodegenerative diseases. “Alzheimer’s is a monumental public health crisis. It’s really important […] to get […] tissues digitized, organized, and made available to scientists and computational people.”
- Bruce J. Darrow, MD-PhD, who leads Mount Sinai’s AI ethics committee, often works on creating spaces and treatments that are not only safe and effective, but also equitable, being tested across the right demographics and taking into account factors such as insurance coverage, income, and zip code.
- Robert Freeman, RN, MSN, NE-BC, is working on moving from the reactive to the predictive and preventative AI. In practice, this would lead to shorter patient time in the hospital and improved overall 30-day mortality.
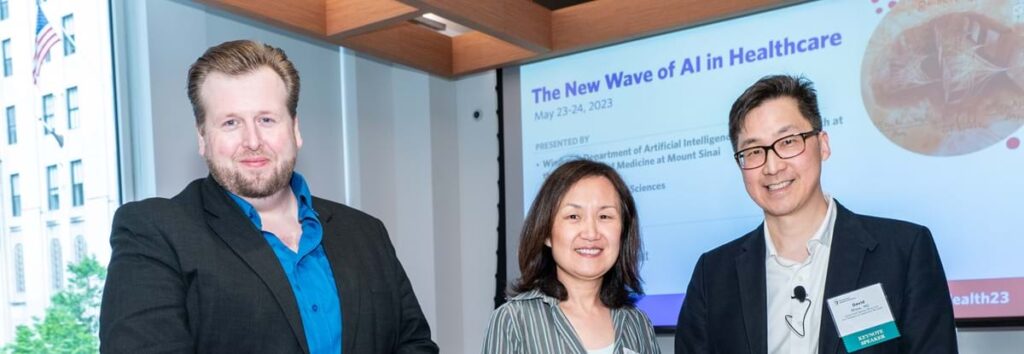
(From left to right) Thomas J. Fuchs, Dr.sc., Mount Sinai Health System, Keynote Speakers: Jianying Hu, PhD, IBM Fellow, Director, HCLS Research, Global Science Leader, AI for Healthcare at IBM Research, David Rhew, MD, Global Chief Medical Officer, Vice-President of Healthcare, Microsoft
Governance and Ethics for the Use of Large Healthcare Datasets for AI
The use of massive amounts of patient data for AI naturally raises key governance and ethics issues, including data privacy, transparency, regulatory compliance, and bias screening to ensure fair representation. These issues and more were covered in a panel discussion moderated by Dean Eric Nestler, MD, PhD, Icahn School of Medicine at Mount Sinai.
The role of bias in large healthcare data models can take many forms, especially when it comes to populations of color and members of underrepresented communities. One of the panelists, Emma Benn, DrPH, a biostatistician at Mount Sinai who applies her training to health equity research, addressed the governance and ethics of the topic. In terms of race, she posed the question of whether the algorithms and technology are just describing racial and ethnic differences versus being able to operationalize race in a way that gets closer to identifying mutable targets for intervention.
“If we’re not operationalizing things correctly, we’re not going to be able to use AI to reduce health inequalities,” says Benn.
Another panelist, Dr. Erik Lium, PhD, discussed the importance of data privacy. Dr. Lium is the Chief Commercial Innovation Officer for the Mount Sinai Health System and the President of Mount Sinai Innovation Partners. One question posed by the audience was which processes a hospital uses to protect a patient’s personal health data. The answer depends on who is going to use said data, be it internally for research or with an external partner. Internally, an institutional review board decides acceptable usage. For use with external partners, it involves legal agreements with copious protections that bar anyone from taking, for instance, de-identified data and attempting to re-identify the data.
“You can use the data for a stated purpose that we think is ultimately beneficial to patients. If you go outside of that purpose, then you’re doing something that’s inappropriate,” says Dr. Lium.
Keynote speaker Dr. David Rhew ended his address on a key philosophical and ethics-focused note, bringing up the idea of AI taking a pause to prevent potentially harmful aspects such as the spreading of misinformation. “If the good actors pause on this, that doesn’t mean that the bad actors are going to pause.”
In Conclusion
Throughout the symposium, speakers were able to address successes, challenges, and future initiatives needed to further the development of new AI technology in the field and how it can be implemented to better patient outcomes. Some of the key takeaways from the symposium’s sessions include:
- Increasing cooperation between institutions.
- Figuring out how to obtain and efficiently process ever larger data sets.
- Using AI to enhance patient experience and outcomes.
Finally, these developments will need to be carefully and continuously filtered through the lenses of equitability and security to ensure that every patient receives the highest level of care no matter the setting. The ‘New Wave of AI in Healthcare’ symposium was an important step towards this equitable, AI-integrated future, with more advancements and discussions to come.
Warning: Undefined variable $showit in /var/www/nyas_develop/nyas/public/wp-content/themes/nyas-theme-child/includes/shortcodes.php on line 1802