How AI is Reshaping Healthcare
The next decade will be a pivotal one for the integration of AI and Big Data into healthcare, bringing both tremendous advantages as well as challenges.
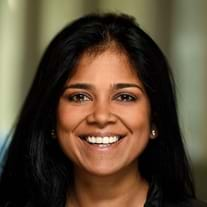
One of the most common causes of death among hospital patients in the United States is also one of the most preventable — sepsis.
Sepsis symptoms can resemble other common conditions, making it notoriously challenging to identify, yet early diagnosis and intervention are critical to halting the disease’s rapid progress. In children, for each hour that sepsis treatment is delayed, the risk of death increases by as much as 50 percent.
Novel innovations, such as the one pioneered by Suchi Saria, director of the Machine Learning and Healthcare Lab and the John C. Malone Assistant Professor at Johns Hopkins University, are helping to reverse this trend. In 2013, Saria and a team of collaborators began testing a machine learning algorithm designed to improve early diagnosis and treatment of sepsis.
Using troves of current and historical patient data, Saria’s artificial intelligence (AI) system performs real-time analysis of dozens of inpatient measurements from electronic health records (EHRs) to monitor physiologic changes that can signal the onset of sepsis, then alert physicians in time to intervene.
“Some of the greatest therapeutic benefits we’re going to see in the future will be from computational tools that show us how to optimize and individualize medical care,” Saria said. She explained that the emergence of EHRs, along with the development of increasingly sophisticated AI algorithms that derive insights from patient data, will fuel a seismic shift in medicine — one that merges “what we are learning from the data, with what we already know from our best physicians and best practices.”
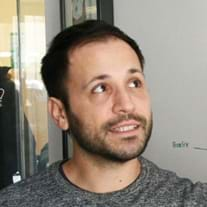
EHRs have become a data gold mine for computer scientists and other researchers who are tapping them in ways designed to improve physician-patient encounters, inform and simplify treatment decisions, and reduce diagnostic errors. Like many other technological advances, though, there are those physicians who regard EHR systems with less enthusiasm.
A 2016 American Medical Association study revealed that physicians spend nearly twice as much time engaged in EHR tasks than they do in direct clinical encounters. Physician and author Atul Gawande recently lamented in The New Yorker that “a system that promised to increase my mastery over my work has, instead, increased my work’s mastery over me.”
Yet, data scientist Nicholas Tatonetti, the Herbert Irving Assistant Professor of Biomedical Informatics at Columbia University envisions a day when such AI algorithms will enable physicians to deepen their interaction with patients by freeing them from the demands of entering data into the EHR. Tatonetti has designed a system using natural language processing algorithms that takes accurate notes while physicians talk with patients about their symptoms. Like Saria’s AI system, Tatonetti’s takes advantage of the vast amount of data captured in EHRs to alert physicians in real time to potentially dangerous drug interactions or side effects.
Unknown Interactions
Anyone who has filled a prescription is familiar with the patient information leaflet that accompanies each medication, detailing potential side effects and known drug interactions. But what about the unknown interactions between medications?
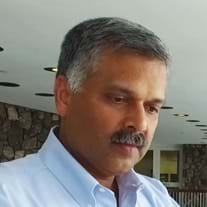
Tatonetti has also developed an algorithm to analyze existing data in electronic health records, along with information in the FDA’s “adverse outcomes” database, to tease out previously unknown interactions between drugs. In 2016, he published a study showing that ceftriaxone, a common antibiotic, can interact with lansoprazole, an over-the-counter heartburn medication, increasing a patient’s risk of a potentially dangerous form of cardiac arrhythmia.
As data-driven AI techniques become more accessible to clinicians, the treatment of conditions both straightforward, like hypertension, and highly complex, such as cancer, will be transformed.
Ajay Royyuru, vice president of healthcare and life sciences research at IBM and an IBM Fellow, explained that, “when a practitioner makes a patient-specific decision, the longitudinal trail of information from thousands of other patients from that same clinic is often not empowering that physician to make that decision. The data is there, but it’s not yet being used to provide those insights.”
In the coming years, physicians and researchers will be able to aggregate and better utilize EHR data to guide treatment decisions and help set patients’ expectations.
The ability to draw on information from tens or even hundreds of thousands of patients, in addition to a physician’s own experience and expertise, could represent a paradigm shift in physician-patient interactions, according to Bethany Percha, assistant professor at the Icahn School of Medicine at Mount Sinai, and CTO of the Precision Health Enterprise, a team that turns AI research into tangible products for the health system.
“Big Data offers us the promise of using data to have a real dialogue with patients — if you’re newly diagnosed with cancer, it means giving people a realistic, data-driven assessment of what their future is likely to be,” she said.
Biases and Pitfalls
Despite the surge of interest and investment in AI over the past two decades, significant barriers to its widespread application and deployment in healthcare remain.
AI systems that tap current and historical patient health data risk reinforcing well-noted biases and embedded disparities. Medical research and clinical trials have long suffered from a lack of both ethnic and gender diversity, and EHR data may reflect patient outcomes and treatment decisions influenced by race, sex or socioeconomic status. AI systems that “learn” from datasets that include these biases will inherently share and perpetuate them.
Percha noted that greater transparency within the algorithms themselves — such as systems that learn which features an algorithm uses to make a prediction — could alert users to obvious examples of bias. Removing bias from AI algorithms is a work in progress, but the research community’s awareness of the issue and efforts to address it mirror a greater push to eliminate bias and decrease inequities in medicine overall. Optimistically, Percha noted that Big Data and AI may ultimately help create a more level playing field in healthcare delivery.
“Clinical decisions made on the basis of data have the potential to be much more standardized across different health facilities, so people who are in a rural area, for example, might have access to the same decision-making benefits as someone in a city,” she said.
Ensuring patient data privacy is another hot-button issue. Training artificial intelligence systems requires access to massive troves of patient data. Despite the fact that this information is anonymized, some patient advocates and bioethicists object to this access without explicit permission from the patients themselves.
Another privacy issue looms equally large: how to safely collect and protect the streams of potentially useful health data generated by wearable devices and in-home technologies without making patients and consumers feel, in Royyuru’s words, “like they are living their lives in front of a camera.” Studies have shown that data from smartphone apps can provide valuable information about the progression of certain diseases, such as Parkinson’s.
Wearables and in-home IoT devices can also extend the realm of clinical observation well beyond the doctor’s office, revealing, for example, important details about a Parkinson’s patient’s ability to complete the tasks of daily living. Yet Royyuru emphasizes that unless patients trust that their data will be kept private and ethically utilized, these technologies will fizzle long before they’re widely adopted.
Building Trust
The next decade will be a pivotal one for the integration of AI and Big Data into healthcare, bringing both tremendous advantages as well as challenges. Some applications of AI, such as image recognition, are already especially well-suited to healthcare — AI algorithms often match or even outperform radiologists in interpreting medical images — while others are far from ready for widespread use.
Saria, who has deployed her system successfully at multiple hospitals says, “physicians often greet news of AI breakthroughs with skepticism because they’re being over-promised results without clear data demonstrating this promise. True integration and adoption of AI requires not just careful attention to physician workflows, but transparency into exactly how and why an algorithm has arrived at a particular recommendation.”
Rather than replacing or challenging a physician’s place in the healthcare ecosystem, Saria believes that AI has the ability to lighten the load, and as algorithms improve, generate diagnostic and treatment recommendations that physicians and patients can both deem trustworthy.
“We are still figuring out how to make real-time information available so that it’s possible for physicians or expert decision-makers to understand, interpret and determine the right thing to do — and to do that in an error-free way, over and over again,” Saria said. “It’s a high-stakes scenario, and you want to get to a good outcome.”
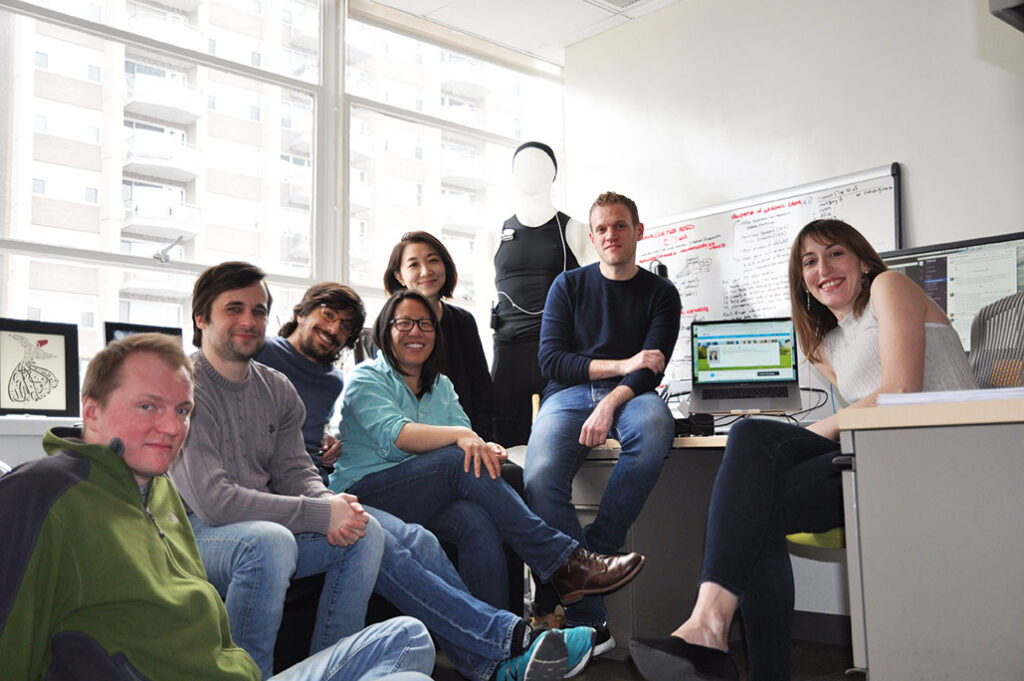
Warning: Undefined variable $showit in /var/www/nyas_develop/nyas/public/wp-content/themes/nyas-theme-child/includes/shortcodes.php on line 1802